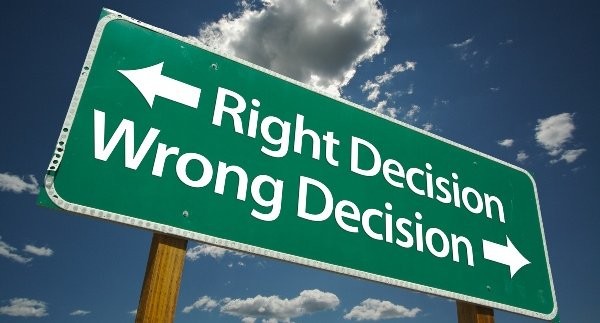
The big data conundrum is one that bedevils most industries, but none more than life sciences. Because of the high stakes of healthcare, there is a great responsibility to get things right, and to pursue continual improvement, ideally with the proper use and analysis of data. In addition, life sciences companies are under more scrutiny and regulation than ever. With more data to collect than any other industry: disease states, scientific studies, individual patient info, clinical results and more, all of that data needs to be turned into actionable information in an increasingly complex digital world.
Undertaking a big data strategy really means that a company is ready to become more “data-driven.” Simply put, that means using more sources of data in order to gain relevant insights to make better decisions and take actions that yield better outcomes. Most successful companies are right more often than not – that’s why they are able to thrive and have a growing business. However, the competitive and regulatory landscape dictates that companies need to “be right faster” and a strategy that incorporates big data requires a serious look at their data management technologies, practices and how they can truely leverage new sources of data.
BIG DATA SHOULD NOT BE JUST A RESEARCH PROJECT
Many companies think big data is a research project, and mistakenly spawn or create a team tasked with testing and evaluating the latest “free” open source technologies such as Hadoop. It’s a cliche, but you can’t select a big data technology, then find a problem to solve. To be successful you must be aligned to a business problem that needs to be addressed. If that problem has a hard deadline, even better! Nothing spells focus like working towards a milestone, and the expectations of frontline business teams that want value immediately. Fortunately with the right modern data management platforms, many business challenges can be solved in weeks, not months or years.
Data governance and stewardship for example is a must. Most companies forget the discipline of trusted, secure and reliable data when they embark upon their big data strategy. The refrain of “the type of data we are capturing in big data projects don’t need governance.” This is a slippery slope. Big data lakes will turn into big data swamps if rigor, process and data quality are not applied. Worse, the insights that are derived from unreliable data are worth less than having no data at all.
At Reltio, we’ve seen our customers use data from traditional 3rd party vendors, and also bringing together public data sources from CMS.gov, Pubmed, clinicaltrials.gov, as well as social media data from Linkedin and Facebook. IoT (internet of things) data, which has the extreme big data volumes at velocity has so far been less of a concern for life sciences companies. In healthcare, health monitoring devices such as the Apple Watch will start to deliver information for physicians from patients that may eventually become part of their care. The trick is to bring capabilities together in a single platform, where data can be correlated, made reliable and for insights to be derived.
For the most part companies have been “playing” with big data technologies, using Hadoop, NoSQL databases, data scientist visualization tools. A lot, and I mean a lot, of money has been spent on pilots and trials. While there have been some successes, for the most part many companies are still immature in their use of these technologies. There are many reasons for this including the IT skills and expertise required to implement new big data tools, and the complexity of integrating data with traditional approaches and applications. Without a singular focus on the desired business outcome, and actual data-driven business applications that are mobile, collaborative and easy to use by frontline sales, marketing and compliance teams, companies will continue to see limited success with big data.
Insights gained run the gamut across healthcare and life sciences and include true 360-degree views and inter-relationships between HCP, HCOs, IDNs, ACOs, MCOs, plans, payers, products, patients and all of their interactions. There are many macro-level conclusions that can be drawn about overall operating efficiency (in the case of commercial operations), and additional data for clinical trials (in the case of R&D). But ultimately the insights derived are only relevant to what can be done with them, and that use is relative to the role and business goals of each user.
DATA MANAGEMENT IS THE APPETIZER. INSIGHTS ARE THE MAIN COURSE
For all of the data management technology and visualization tools invested in bringing together and processing big data, companies are typically left to their own devices, to draw their own conclusions from the insights, and then to act upon them. New data- driven applications are able to synthesize that information and provide suggestions or recommended actions to the frontline business users that are actionable occur daily in the consumer world. Take LinkedIn for example. It brings together vast quantities of data, and delivers suggestions to you. LinkedIn suggests jobs that are relevant to you and your experience. It doesn’t just say here’s a pool of jobs, and makes you go filter and search for the ones that are relevant to you. It understands complex connections and relationships, and shows you the best path to connect to people you don’t know. Business teams, such as sales and account managers in life sciences, need similar help in their day-to-day operations. But they are saddled with legacy CRM and process- driven applications that capture data, but do not offer recommendations and suggestions gleaned from processing large amounts of data and relating them together.
In another simple example, a data-driven application for a pharma sales rep should provide a recommended best path to connect with a key influencer in a formulary committee. Or it might guide a marketing professional to the best candidates for key opinion leaders (KOL) for events. As data-driven applications become more mainstream in our everyday lives as consumers, business users are coming to expect the same degree of capabilities in their day-to-day applications.
SHARING IS CARING
Contrary to popular belief, big data is more than just about size. We’ve all heard about the 3 Vs of volume, velocity and variety, but one key “V” not discussed often enough is veracity. Simply put, that means data quality. Data that is not cleansed and continuously managed cannot be related together for insights. For people, not seeing data in a shared central pool is often a problem. Siloed data, no matter the size, causes issues. Different perspectives of the same customer, product or organization mean collaboration is not possible. Shared insight is as valuable as insight derived from the volume of big data that is now available. From a process perspective, companies need to manage and secure their new-found big data. Having valuable insights is competitive advantage. Many companies simply do not have the compliance and regulatory controls to protect their own data, or meet mandated guidelines such as HIPAA.
In conclusion whether Big Data in life sciences is a blessing or curse depends on whether the organization repeats the same mistakes, or proceeds down the wrong path to obtain much desired insight. Mistakes to avoid include:
• Not ensuring the data is reliable as a foundation. Either ignoring it or making it someone else’s responsibility. This is why master data management (MDM) is a siloed billion dollar industry that hasn’t yielded expected results. Most new data-driven apps have MDM built-in
• Using visualization tools and business intelligence to analyze big data to derive one-time high-level macro insights, but not having an integrated strategy or technology to execute on those insights
• Forgetting about the end business user. It’s great to get lots of data and process in, but time to value and putting it into the hands of the business user in mobile, easy-to-use applications is often last on the list, when it should be the first
• Gathering all the data they can, just because they can. Relevant data and insights that yield recommended actions don’t mean capturing the entire universe. A data sourcing plan is critical to determining what data is relevant and how you are going to leverage it. It’s okay to start small, then increase to big data volumes. A modern data management platform offers the ability to incrementally add data sources, without having to re-architect and start over.
• Not closing the loop or measuring the benefits once you obtain insights, and take action. Continuous monitoring and correlation of insights to action to measure ROI and to allow machine learning systems to use historical context to predict trends is one of the biggest gaps in siloed, disparate tools today. Modern data management platforms provide a complete integrated loop that delivers reliable data, relevant insights and recommended actions that support IT and deliver data-driven applications to business users.